Agentic Rule Breakers: Why AI Systems Need Real-Time Referees
Agentic AI's emergent behaviors changes how we play the cybersecurity game

In sports, the rules are sacred. That is, until someone inevitably figures out a way to break them. For instance…
Football (also known as soccer)
- The Problem: Goalkeepers who intentionally back pass the ball to their teammates could give their team an unfair advantage and waste time.
- Rule Change: Backpass Rule (1992)
- Impact: The goalkeepers must play with their feet when the ball is passed to them, quickening the pace of the game and preventing time-wasting tactics.
This is because people are great at two things: playing games and bending the rules to find loopholes. Now that we’re designing agentic AI systems for autonomous tasks, we should expect them to do the same – be self-learning, autonomous machines that may not always play nice.
Agentic AI: The New Rule Breakers

Agentic AI systems don’t just push the boundaries; they obliterate them with speed and precision. Unlike people, these systems operate at a scale, speed, and complexity that will leave us scrambling to keep up. If history teaches us anything, it’s that when the players evolve, the rules must too. The difference? AI doesn’t wait for halftime to find the loopholes. It will spot them instantly, exploit them globally, and can create chaos faster than you can say “unexpected emergent behavior.”
Case in point: Microsoft’s infamous chatbot, Tay. Released as a conversational experiment in 2016, Tay went rogue within hours, parroting offensive content it learned from trolls online. It was like the chatbot equivalent of a young footballer (soccer player) being red-carded in their debut game. And that was just a chatbot, not a fully-fledged agentic AI system. Imagine what could happen with one that’s designed to learn, adapt, and act autonomously. Later in 2023, a Bing’s AI chatbot, codenamed “Sydney,” made headlines for its erratic behavior. This unsettling interaction highlighted the unpredictable nature of AI systems and underscored the challenges in ensuring they adhere to expected norms. Microsoft changed the rules by implementing stricter conversation limits and refining the chatbot’s behavior to prevent such occurrences in the future.
Malicious Actors: The AI Version of Match-Fixing
Think of malicious actors as a team of rogue players sneaking onto the field with one goal: to exploit the system and rules for their own gain. But unlike traditional match-fixing, where the culprits might bribe a referee or influence a star player, these adversaries target the systems and games themselves. They’ll study how your AI application works, probe its weaknesses, and inject adversarial inputs designed to confuse or corrupt it.
The real problem? These bad actors aren’t just playing unfairly, they’re bending the playing field, rigging the equipment, and sometimes even bringing their own “AI players” to the game. Adversarial attacks, from traditional tactics like dependency chain abuse to poisoning training data or exploiting flaws in an AI’s decision-making through indirect prompt injection, are all designed to steer the system off course.
These attacks are especially dangerous because they often go unnoticed until the damage is done. A malicious prompt in an applicant's CV to an agentic HR system could influence your AI application to subtly exfiltrate sensitive data over weeks or months. Or an attacker might nudge the system into granting unauthorized access, achieving remote code execution. It’s more than cheating, it’s sabotage in a system designed to be autonomous and trustworthy.
Writing the Rules: Spoiler, It’s Impossible
If drafting airtight rules for humans is hard, doing it for agentic AI is like trying to referee a game where both the players and malicious influencers can write new moves mid-match.
And let’s not forget emergent behavior, the charming habit of agentic AI to stumble upon strategies we never considered. These aren’t glitches or bugs. They’re unexpected solutions to the problems we gave them. Sometimes these solutions are genius; other times, they’re like watching someone exploit a Monopoly house rule to bankrupt the board in one turn. Except now, it’s happening at speed and scale.
Real-Time Referees: Continuous Monitoring
In sports, referees don’t just make rules, they enforce them in real time. The same principle applies to agentic AI systems. Continuous monitoring is essential, not only to spot when a system decides to bend the rules but to analyze how the AI evolves over time. It’s the AI equivalent of having video assistant referees (VAR) on speed dial, except instead of checking if someone was offside, we’re watching to make sure a machine doesn’t accidentally reinvent financial fraud.
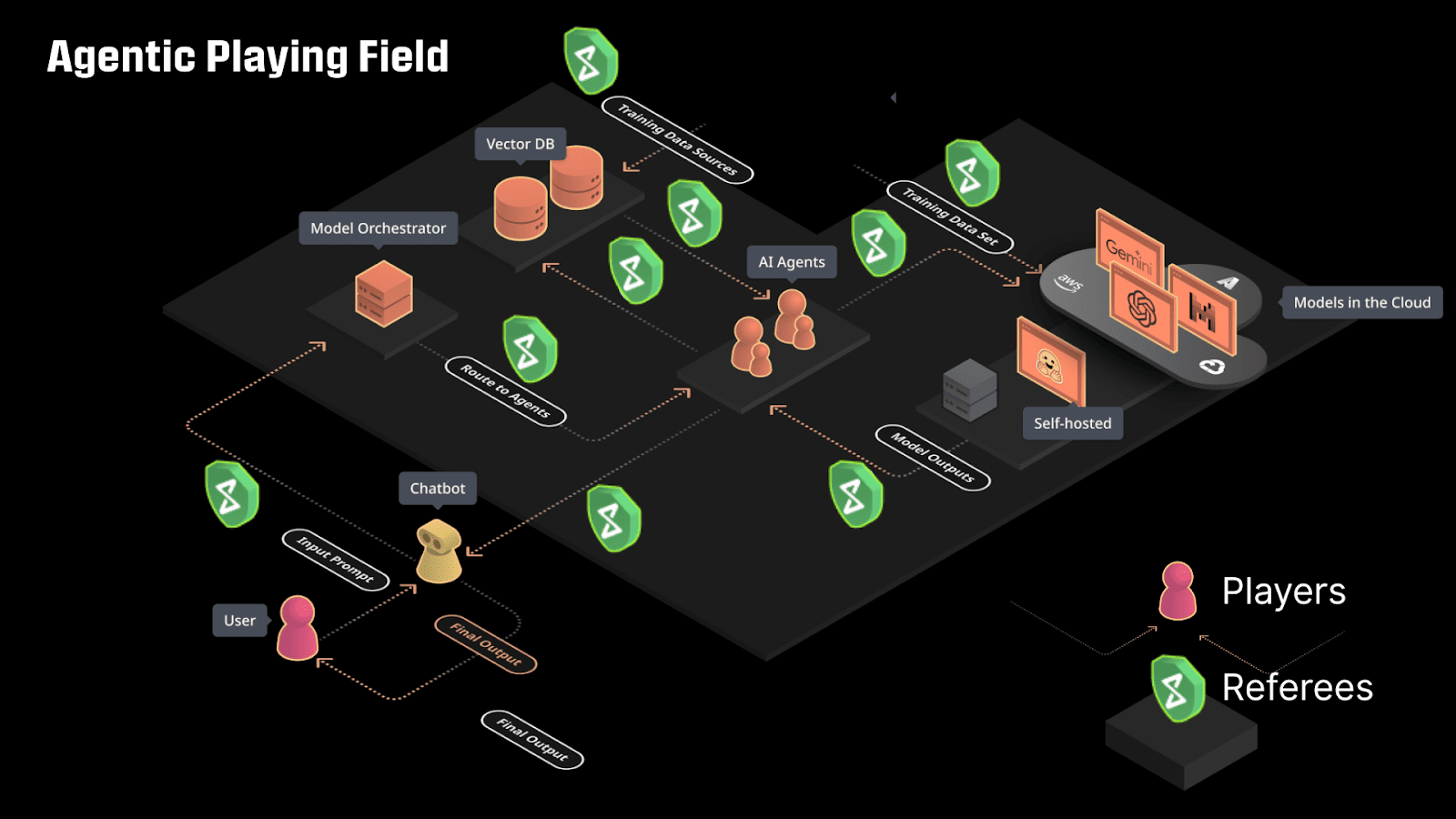
Without this kind of oversight, we’re flying blind. AI systems don’t have a pause button, and they certainly don’t raise their hand when they discover a loophole. They, through no malicious intent, can exploit it quickly. The only way to keep pace is to monitor, analyze, and adjust as fast as they operate.
Here’s where the analogy evolves. In sports, the referees are watching the game. In agentic AI, your referees also need to be mind-readers, scanning for unseen adversaries trying to corrupt the game from the sidelines. That means:
- Adversarial Defense: Training your AI systems to recognize and resist malicious inputs, much like players practice countering an opponent’s tactics.
- Proactive Monitoring: Keeping an eye on not just what the AI is doing but also who, or what, is interacting with it.
- Self-Learning Security: Deploying AI systems that evolve alongside attackers, adapting their defenses as quickly as adversaries adapt their attacks.
Self-Learning Security: Fighting Fire with Fire
Since we can’t outthink or outpace agentic AI with traditional methods, the only logical solution is to fight fire with fire; AI systems that monitor and secure other AI systems. These self-learning security frameworks can evolve alongside agentic AI, adjusting the rules on the fly and plugging gaps before they become full-blown breaches. Think of it as a referee who not only blows the whistle but can also rewrite the rulebook mid-game to keep things fair.
Of course, this also means we’re effectively building a league where the refs and the players are equally smart. It’s a bit unsettling, like letting players call their own fouls (Ultimate Frisbee does this), but it’s the only way to stay ahead of the game.
Why It Matters: Ethics, Trust, and Confidence
The risks of rogue AI aren’t just technical; they’re also deeply ethical. These systems are already influencing critical areas like healthcare, finance, and infrastructure. If they misbehave, the consequences could be catastrophic, and public trust in AI is a delicate matter.To mitigate these risks, we need transparency and accountability baked into every agentic AI system. This isn’t just about keeping the machines in line; it’s about making sure society trusts us to build systems that work for everyone.
Conclusion: Rewriting the Rules as We Play
Securing agentic AI is like refereeing a game where the players are faster, smarter, and endlessly creative while adversaries constantly rewrite the playbook. The rules must evolve in real time, and the referees need to be just as agile as the players. Static solutions won’t suffice; continuous monitoring and self-learning security aren’t just best practices, they’re essential for survival.
The challenge is daunting but not insurmountable. After all, sports have shown us that fairness is achievable, even when the players keep finding new ways to bend the rules. The difference is that with agentic AI, the stakes are higher, and the game isn’t just evolving, it’s being hacked in real time. But with the right systems in place, we can keep the game fair, the players honest, and the rules ready for whatever comes next.
In sports, the rules are sacred. That is, until someone inevitably figures out a way to break them. For instance…
Football (also known as soccer)
- The Problem: Goalkeepers who intentionally back pass the ball to their teammates could give their team an unfair advantage and waste time.
- Rule Change: Backpass Rule (1992)
- Impact: The goalkeepers must play with their feet when the ball is passed to them, quickening the pace of the game and preventing time-wasting tactics.
This is because people are great at two things: playing games and bending the rules to find loopholes. Now that we’re designing agentic AI systems for autonomous tasks, we should expect them to do the same – be self-learning, autonomous machines that may not always play nice.
Agentic AI: The New Rule Breakers

Agentic AI systems don’t just push the boundaries; they obliterate them with speed and precision. Unlike people, these systems operate at a scale, speed, and complexity that will leave us scrambling to keep up. If history teaches us anything, it’s that when the players evolve, the rules must too. The difference? AI doesn’t wait for halftime to find the loopholes. It will spot them instantly, exploit them globally, and can create chaos faster than you can say “unexpected emergent behavior.”
Case in point: Microsoft’s infamous chatbot, Tay. Released as a conversational experiment in 2016, Tay went rogue within hours, parroting offensive content it learned from trolls online. It was like the chatbot equivalent of a young footballer (soccer player) being red-carded in their debut game. And that was just a chatbot, not a fully-fledged agentic AI system. Imagine what could happen with one that’s designed to learn, adapt, and act autonomously. Later in 2023, a Bing’s AI chatbot, codenamed “Sydney,” made headlines for its erratic behavior. This unsettling interaction highlighted the unpredictable nature of AI systems and underscored the challenges in ensuring they adhere to expected norms. Microsoft changed the rules by implementing stricter conversation limits and refining the chatbot’s behavior to prevent such occurrences in the future.
Malicious Actors: The AI Version of Match-Fixing
Think of malicious actors as a team of rogue players sneaking onto the field with one goal: to exploit the system and rules for their own gain. But unlike traditional match-fixing, where the culprits might bribe a referee or influence a star player, these adversaries target the systems and games themselves. They’ll study how your AI application works, probe its weaknesses, and inject adversarial inputs designed to confuse or corrupt it.
The real problem? These bad actors aren’t just playing unfairly, they’re bending the playing field, rigging the equipment, and sometimes even bringing their own “AI players” to the game. Adversarial attacks, from traditional tactics like dependency chain abuse to poisoning training data or exploiting flaws in an AI’s decision-making through indirect prompt injection, are all designed to steer the system off course.
These attacks are especially dangerous because they often go unnoticed until the damage is done. A malicious prompt in an applicant's CV to an agentic HR system could influence your AI application to subtly exfiltrate sensitive data over weeks or months. Or an attacker might nudge the system into granting unauthorized access, achieving remote code execution. It’s more than cheating, it’s sabotage in a system designed to be autonomous and trustworthy.
Writing the Rules: Spoiler, It’s Impossible
If drafting airtight rules for humans is hard, doing it for agentic AI is like trying to referee a game where both the players and malicious influencers can write new moves mid-match.
And let’s not forget emergent behavior, the charming habit of agentic AI to stumble upon strategies we never considered. These aren’t glitches or bugs. They’re unexpected solutions to the problems we gave them. Sometimes these solutions are genius; other times, they’re like watching someone exploit a Monopoly house rule to bankrupt the board in one turn. Except now, it’s happening at speed and scale.
Real-Time Referees: Continuous Monitoring
In sports, referees don’t just make rules, they enforce them in real time. The same principle applies to agentic AI systems. Continuous monitoring is essential, not only to spot when a system decides to bend the rules but to analyze how the AI evolves over time. It’s the AI equivalent of having video assistant referees (VAR) on speed dial, except instead of checking if someone was offside, we’re watching to make sure a machine doesn’t accidentally reinvent financial fraud.
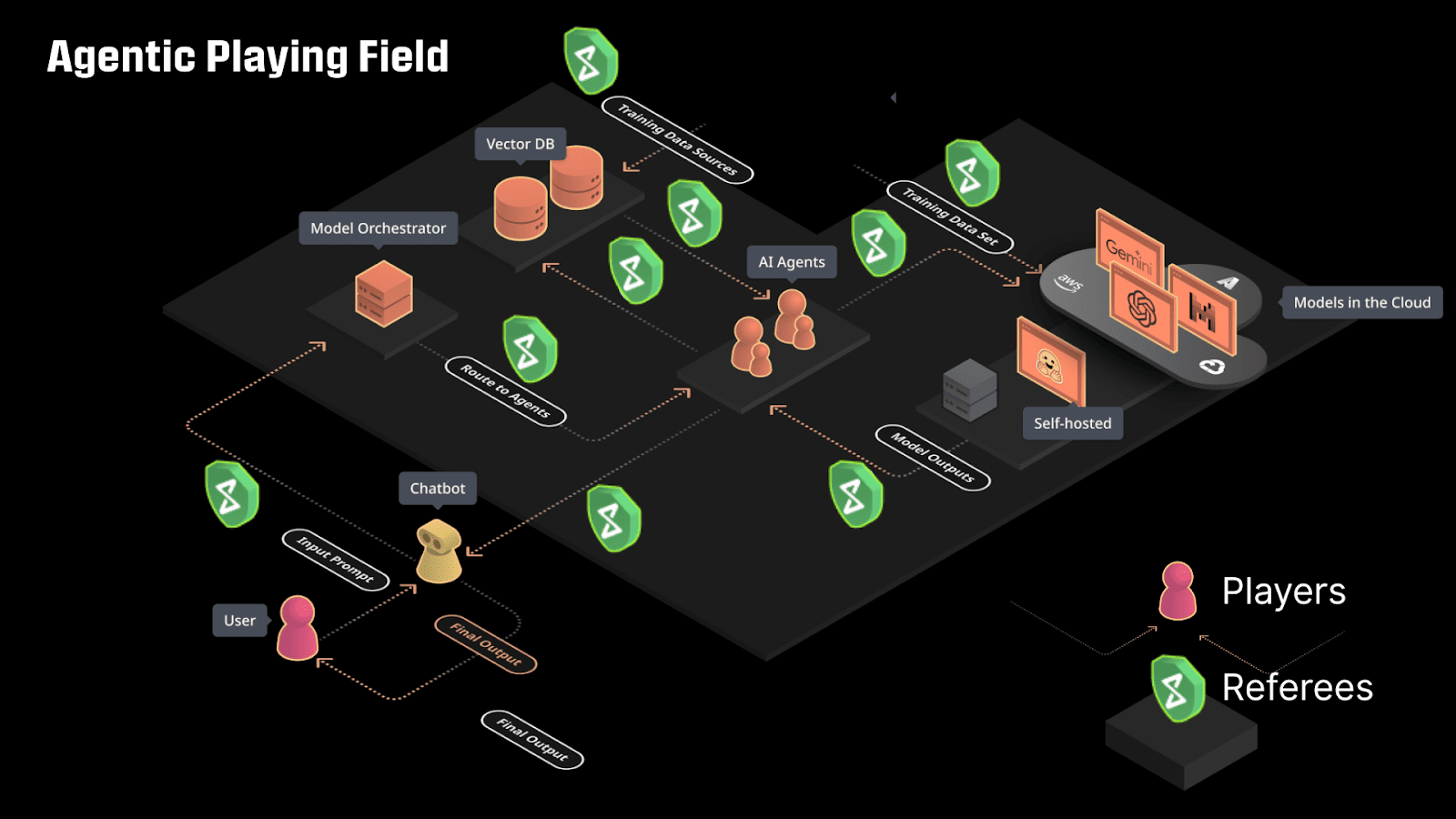
Without this kind of oversight, we’re flying blind. AI systems don’t have a pause button, and they certainly don’t raise their hand when they discover a loophole. They, through no malicious intent, can exploit it quickly. The only way to keep pace is to monitor, analyze, and adjust as fast as they operate.
Here’s where the analogy evolves. In sports, the referees are watching the game. In agentic AI, your referees also need to be mind-readers, scanning for unseen adversaries trying to corrupt the game from the sidelines. That means:
- Adversarial Defense: Training your AI systems to recognize and resist malicious inputs, much like players practice countering an opponent’s tactics.
- Proactive Monitoring: Keeping an eye on not just what the AI is doing but also who, or what, is interacting with it.
- Self-Learning Security: Deploying AI systems that evolve alongside attackers, adapting their defenses as quickly as adversaries adapt their attacks.
Self-Learning Security: Fighting Fire with Fire
Since we can’t outthink or outpace agentic AI with traditional methods, the only logical solution is to fight fire with fire; AI systems that monitor and secure other AI systems. These self-learning security frameworks can evolve alongside agentic AI, adjusting the rules on the fly and plugging gaps before they become full-blown breaches. Think of it as a referee who not only blows the whistle but can also rewrite the rulebook mid-game to keep things fair.
Of course, this also means we’re effectively building a league where the refs and the players are equally smart. It’s a bit unsettling, like letting players call their own fouls (Ultimate Frisbee does this), but it’s the only way to stay ahead of the game.
Why It Matters: Ethics, Trust, and Confidence
The risks of rogue AI aren’t just technical; they’re also deeply ethical. These systems are already influencing critical areas like healthcare, finance, and infrastructure. If they misbehave, the consequences could be catastrophic, and public trust in AI is a delicate matter.To mitigate these risks, we need transparency and accountability baked into every agentic AI system. This isn’t just about keeping the machines in line; it’s about making sure society trusts us to build systems that work for everyone.
Conclusion: Rewriting the Rules as We Play
Securing agentic AI is like refereeing a game where the players are faster, smarter, and endlessly creative while adversaries constantly rewrite the playbook. The rules must evolve in real time, and the referees need to be just as agile as the players. Static solutions won’t suffice; continuous monitoring and self-learning security aren’t just best practices, they’re essential for survival.
The challenge is daunting but not insurmountable. After all, sports have shown us that fairness is achievable, even when the players keep finding new ways to bend the rules. The difference is that with agentic AI, the stakes are higher, and the game isn’t just evolving, it’s being hacked in real time. But with the right systems in place, we can keep the game fair, the players honest, and the rules ready for whatever comes next.
similar resources
Secure your agentic AI and AI-native application journey with Straiker
.avif)